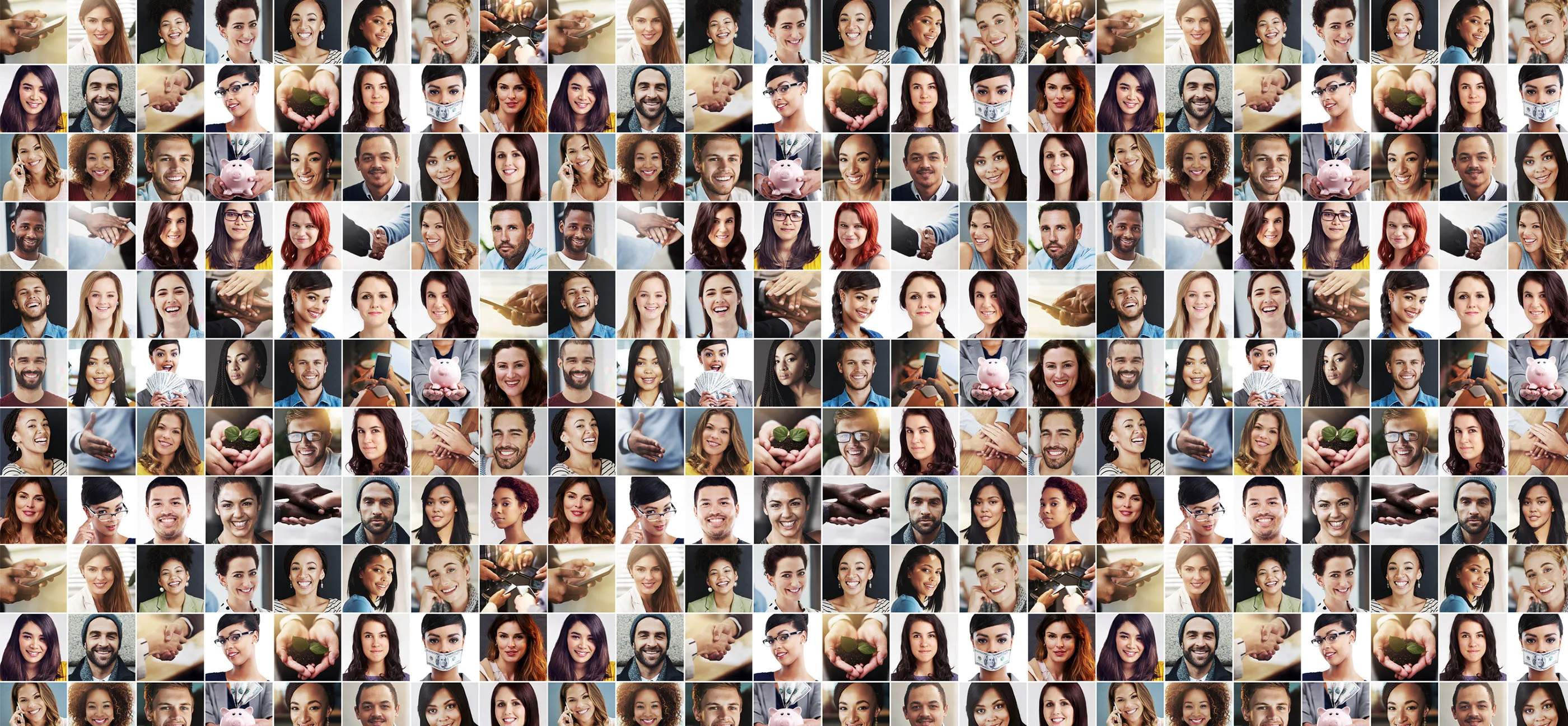
Most companies rely on artificial intelligence-based algorithms to make a wide variety of business decisions—from pinpointing the products customers prefer to determining which resumes should go to hiring managers.
The problem for companies trying to advance racial equity and inclusion: Algorithms can amplify bias.
Some companies try to address the issue by making sure that their algorithms don’t use data on protected characteristics such as race or gender. Yet, eliminating factors like race from an algorithm doesn’t address the problem. Rather, doing so might even magnify the issue.
Instead, in a recent article in Proceedings of the National Academy of Sciences, Harvard Business School professors Eva Ascarza and Ayelet Israeli suggest intervening at points along the algorithm’s decision-making chain using what they call “bias-eliminating adapted trees” (BEAT) to ensure that the algorithm generates fair outcomes. As the algorithm sorts through information to optimize its objective, BEAT detects and eliminates bias at key points in the training process. For instance, BEAT could help a car service charge surge pricing during rush hour without discriminating against race or other sociodemographic characteristics.
HBS Working Knowledge spoke with Israeli, the Marvin Bower Associate Professor of Business Administration, and Ascarza, the Jakurski Family Associate Professor of Business Administration, about how managers can apply the findings to eliminate discriminatory business practices.
Rachel Layne: People may assume that algorithms are unbiased, but that’s not always the case. Where does bias creep in?
Ayelet Israeli: There are several sources of algorithmic bias. One is due to historical human bias. When you train your algorithm, you use historical data that might have biases, and these will continue. For instance, a few years ago, Amazon used an algorithm to help them decide who to employ. And the algorithm disproportionally picked men over women because historically, they had hired more men than women. Because humans made decisions that were biased, the algorithm perpetuates this.
Another source of bias is incomplete or unrepresentative information. A famous example is facial recognition. If I use mostly photos of white men to train the machine to learn facial recognition, the machine will perform poorly on recognizing people of color and women.
In our article we're actually focusing on a third type: Even if the data used to train the algorithm is unbiased and representative, there may be correlations between the person’s protected attributes and their behaviors or features that are captured in the data, which may lead to biased outcomes.
Layne: Can you give an example?
Israeli: Suppose that women are more likely to buy red iPhone cases compared to other groups. Now, I decide to exclude gender when I train my algorithm to predict something, in order to prevent any biased outcome. But if I still let the algorithm use the color of the iPhone case, then I'm essentially using a proxy for “this person is a woman.”
Then, when the algorithm uses the color of the case to decide who to hire or who to give a discount to, the algorithm may favor the group that has red cases, meaning it disproportionally favors women, despite having removed the gender information from the data. And, as decision-makers, we might not want to do that.
Most algorithms do not create the bias per se, but tend to exacerbate it because of scale. And this becomes very problematic.
Layne: If the problem is in the data, are algorithms creating the bias or was it already there? And is it deliberate?
Eva Ascarza: Most algorithms do not create the bias per se, but tend to exacerbate it because of scale. And this becomes very problematic. Think about the three types of sources that Ayelet mentioned. The first type of algorithmic bias takes [pre-existing] human decisions and automates that process. So, instead of a few humans making decisions, now you can have millions of machines making systematically biased decisions. That scale can have a significant impact on society.
The second one is also exacerbating bias at scale. If you're going to start doing facial recognition to evaluate millions of people, even tiny algorithmic mistakes in prediction across races or genders make a huge difference in the aggregate; any decision that is made based on the prediction of those algorithms will inherently include bias.
In the third case, the bias appears because algorithms add great levels of precision thanks to the scale of the data. For example, algorithms help companies, hospitals, and banks predict what individuals will do, and they do so by finding hidden patterns in the data. Compared to a human looking for predictable patterns in simple data, an algorithm is doing it on steroids, using very large datasets, and is able to capture patterns that are undetectable to humans. If you do it on steroids, these correlations [such as the color of the case and the gender] kick in and create systematic differences that now are sizable. Like when people found out that Uber was charging people in poor neighborhoods systematically higher prices than those in richer areas.
Whether it's purposeful or not, we don't know for every company, obviously, but we believe that it’s likely not intended. That said, the problem for the firm is having not been careful to look at the outcomes of these algorithms to detect these biases in the first place.
The positive outlook here is—if you compare algorithmic bias to human bias—with algorithmic bias, you can at least offer ways to solve it, and our solution is one of them.
Israeli: The positive outlook here is—if you compare algorithmic bias to human bias—with algorithmic bias, you can at least offer ways to solve it, and our solution is one of them. Because it's a machine, when you're learning about an issue, you can fix it. Whereas with human bias, we've been trying to deal with it for years. And it's so hard to change. It's so hard to monitor or fix compared to the machine.
Layne: In layman's terms, how does your solution, BEAT, work? You mentioned surge pricing for car services as an example.
Israeli: So, imagine we have three different variables to determine how much the car ride should cost: Time of day, number of passengers, and which neighborhood is the origin or the endpoint.
Let's say that the time of day is not systematically different across people. So, people of all types go at different times of day. Same for the number of passengers. But the third variable, origin and endpoint, systematically varies across people of different groups; people of color tend to live in a certain neighborhood [due to historical disparities].
In general, a pricing algorithm will explore patterns between these three variables and the firm’s profit to see what the firm should charge depending on those characteristics. In contrast, our algorithm will not only explore which price to charge, but also whether each variable we use to determine price creates a biased outcome. For example, the algorithm explores time of day and determines: If it's very late, it should charge more. If it's early, it should charge less. The algorithm will also look for, “Oh, when I split the data by time of day, there is no difference between gender or race. Basically, there's the same type of people in both. So I can follow this rule.”
But when it does the split based on the origin or destination, it will see very quickly, “Oh, if I do the split here, I have mostly people of color” or “here, I have mostly white people.” In those cases, the algorithms ignores that information; it doesn't want to learn things that will impact different groups in different ways. In a way, we only want it to learn the things that are not going to create discrimination. And so the algorithm relies on other variables instead, such as number of passengers, whatever the other things that are not related to race or ethnicity.
Layne: What responsibility do managers have to eliminate bias in the algorithms they deploy?
Israeli: You should at least know what is going on. The number one thing to do is audit your algorithms and check if you have outcomes that are systematically different. And then based on whatever the objective is, whatever your business intentions are, you can decide what to do. But you need to know what is going on. At the very least, our algorithm provides a simple tool to empower managers to do just that, then it can also help them eliminate or reduce that bias if they want to do so.
Ascarza: At the end of the day, it is the firm that should decide how they make their profit. Firms should decide how they grow, and what kind of market they want to be in. But they shouldn't make business decisions blindly, right? That's why we believe this is important to give businesses the tools to audit the outcomes of their algorithms and to make the decision themselves.
You Might Also Like:
Feedback or ideas to share? Email the Working Knowledge team at hbswk@hbs.edu.
Image: iStockphoto/cagkansayin