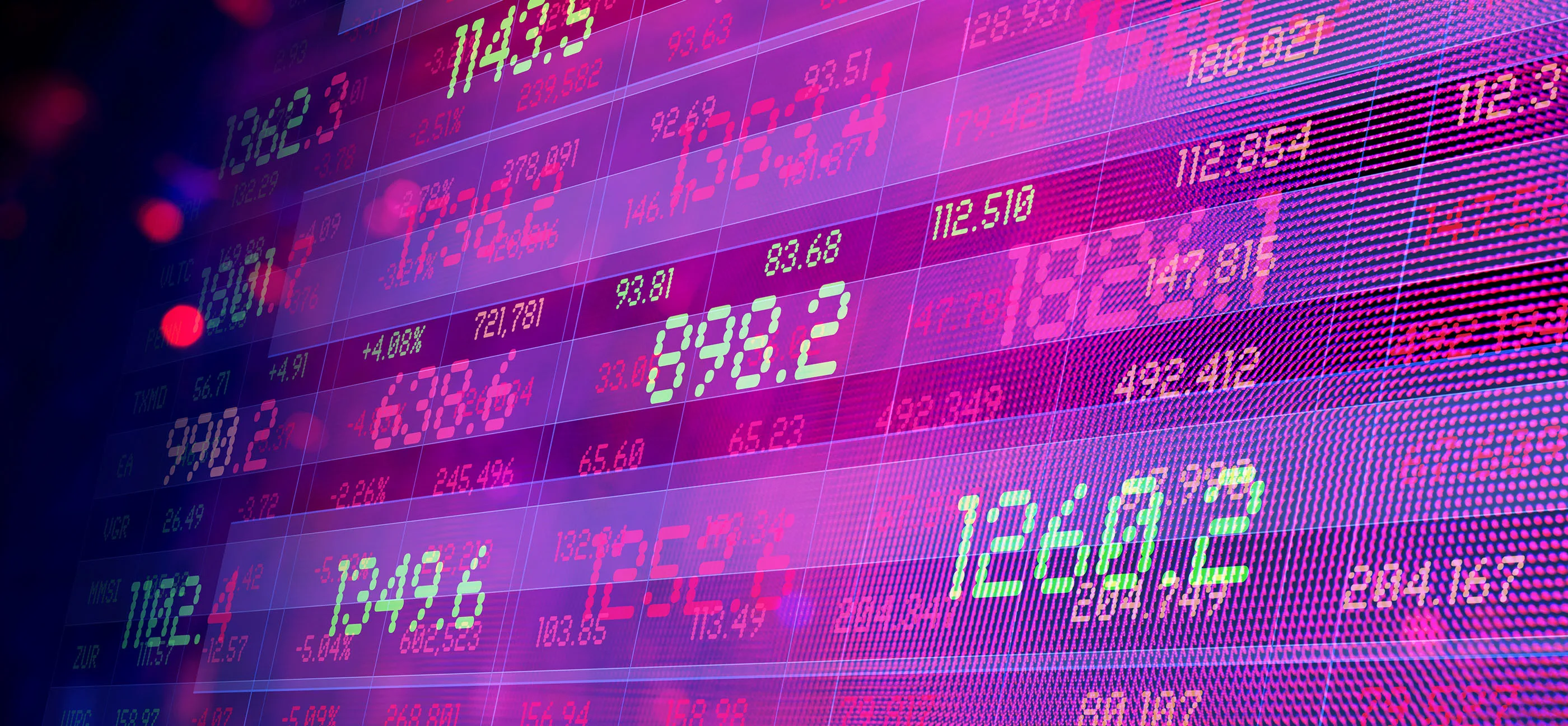
Traditional investing classifications consider Walmart a consumer staples retailer, but the company owns more than 6,000 retail and distribution properties around the world—the portfolio dwarfs those of many commercial real estate firms.
“It was a major discovery to me that Walmart is also a real estate company—they have a lot of buildings,” says MarcAntonio Awada, head of research and data science at the Digital, Data, and Design Institute at Harvard (D^3). For an investor looking for an edge, the distinction could be critical.
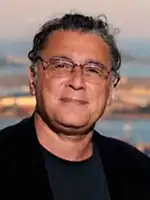
MarcAntonio Awada
Rapid technological change has blurred industry lines as companies increasingly bring seemingly unrelated business lines together in unconventional ways. New research by Awada, Harvard Business School Professor Suraj Srinivasan, and doctoral student Paul J. Hamilton harnesses machine learning and regulatory filings to uncover such nuances at companies.
Portfolio managers and analysts have been using the Global Industry Classification Standard, or GICS—a taxonomy of 11 sectors, 25 industry groups, and additional subsets—to compare stocks since 1999. Periodically, Standard & Poor’s and Morgan Stanley Capital International, the companies that maintain the system, review the categories and how they classify companies.
In the last 20 years, the boundaries of businesses have become much more diffuse because of digital technology driven business models.
In contrast, the researchers used machine learning to analyze company descriptions from 10-K filings dynamically, weighting companies across 15 different “TOPICS” and three tiers. The study, published in February, found that long-short equity portfolios designed using the TOPICS classification approach outperformed those created using GICS categories in risk-adjusted returns for health care, utilities, energy, real estate, and technology by as much as 2.5 percentage points on an annualized basis.
The TOPICS method uses sophisticated financial models to spot hidden similarities in risk and return profiles among seemingly disparate stocks, revealing new investment opportunities. Conversely, the models also highlight opposing profiles within sectors.
We recently talked to Awada and Srinivasan, the Philip J. Stomberg Professor of Business Administration, about the study.
Danielle Kost: Why should the investment world reconsider how it categorizes companies?
Srinivasan: We used to be in the world of conglomerates 30 or 40 years ago, and those changed over time. We started having much more pure-play companies. And so for a period of time, these industry classifications meant something. It was not perfect, but there was no alternative.
In the last 20 years, the boundaries of businesses have become much more diffuse because of digital technology driven business models.
Now, if you think about Walmart and Amazon, both their business models have changed over time. Many companies are becoming tech companies. The difference between Apple and Visa is quickly disappearing when you consider about Apple Pay. Amazon is competing with pharmacy businesses now.
So what defines industry membership of a company? And where does an industry start and where does it end? Parallel to all these is our capacity to understand and use alternate data techniques to assess and understand a business.
We want to make money and always looking to generate alpha in our investment strategies, so if machine learning can make us profitable and improve our risk management and trading efficiency, so be it.
Kost: It seems like machine learning plays an important role here. What has been the attitude toward artificial intelligence within institutional investing? Are investors embracing it? Is there skepticism?
Awada: I can tell you from my previous work [as a hedge fund manager] I think that we’re always on the cutting edge when it comes to using machine learning. The bottom line is that we want to make money and always looking to generate alpha in our investment strategies, so if machine learning can make us profitable and improve our risk management and trading efficiency, so be it.
Srinivasan: It’s definitely being embraced. Machine learning is the latest quantitative technique, but that extends to a lot of other parts of the investing world.
The human-plus-machine idea is making a big impact in how things are being done. There are things that machines can do that humans don’t have to do. But at the end of the day, in many cases we might still want to rely on a human intervention.
So if you're a quantitative trader, you're always improving your models and driving your trading strategies that way. Machine learning is the current version of how to do that really well. In other places where it was maybe more human-based decision-making it's now human-plus-machine-based decision-making.
Kost: Do you see the potential for a shift away from the GICS?
Srinivasan: We develop certain standards because it creates a common way for us to understand and communicate what we are doing. It creates a common set of techniques that everybody can use. And so, unless there is a good alternative, it's hard to drop something. It gets embedded in models, it gets embedded in contracts.
You might have a measurement system that says this fund manager will be paid based on how much the return beats the GICS portfolio returns and that’s locked in, which means if I'm a fund manager, I'm going to get paid based on how much I do better than my GICS segment. I'm then stuck with using the GICS framework. If you tell me we can’t use GICS anymore, you have to tell me what else to benchmark on.
Once I say that the world is more complex, it makes simple solutions harder. How do I benchmark somebody's performance for pay? If the benchmark keeps changing, it complicates several things. So there’s a simple advantage that comes from using standard frameworks.
Awada: I take a much more practical view. In the last five or six years, portfolio returns have suffered, especially in distressed market conditions.
Let’s take COVID 2020. You had certain stocks in certain sectors behaving in a certain way and they were not aligned.
You look at Amazon’s stock price, for example. It was going through the roof, while Walmart was going down. So, if you are a portfolio manager and you are looking at the retail sector, you are puzzled why Amazon is doing well the way it is, and why Walmart is doing poorly the way it is. Well, Amazon is going through the roof because it’s a technology company in many respects—and it’s information technology. It's more than selling books and records and retail online.
So I think that's where a lot of portfolio managers started seeing misalignment in performance among stocks supposedly belonging to the same GICS sector resulting in big losses on their books. This can be attributed to misalignment in the risk profiles of such stocks, and fund managers wanted to hedge that risk. Well, how can I hedge my risk against a portfolio that has retail stocks and technology stocks? If you have put Amazon into the retail, you're not putting the right notional risk allocation towards that sector. So, therefore, the sector classification is becoming critical in terms of the way you manage notional risk.
Kost: How might finance rethink other approaches to benchmarking?
Awada: Well, is the S&P 500 really representative of the market? You know, why 500 stocks? Why can't we have stocks that have a true sense of one sector identification? Using our methodology, it could be 300 of them that really represent the market.
I feel the S&P 500 could have a challenge down the road as an index, in my opinion.
If you look at Europe, the stock or the index that represents the market is the Euro Stock 50. It's not how many stocks, it’s how much they are representative. I feel the S&P 500 could have a challenge down the road as an index, in my opinion, that is even more representative of the market and therefore give investors more of an idea of where they should actually allocate their funding.
Srinivasan: Imagine the stickiness of the S&P 500? There are trillions and trillions of pension assets in S&P funds. Some things are just sticky because they are the benchmark.
You Might Also Like:
Feedback or ideas to share? Email the Working Knowledge team at hbswk@hbs.edu.
Image: iStockphoto/simoncarter